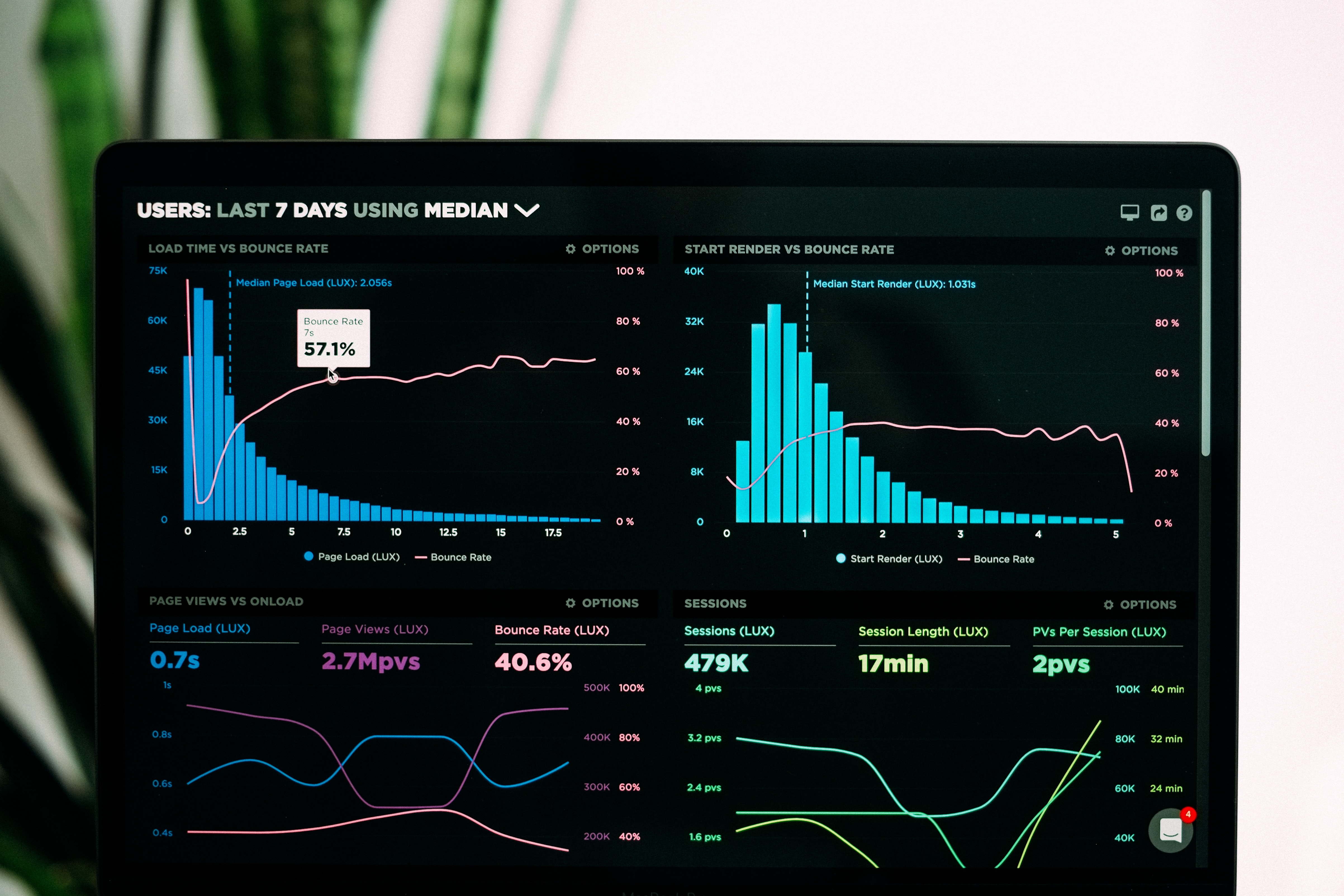
CONTEXT: Fascinating report on research looking at ways to improve the way we can use retrospective data and help avoid selection bias. Using increasingly sophisticated methods to look at all the data in a chart review, in this case delving into the messy data that is the free text, to give the clearest picture possible will surely only enhance the value of RWR.
IMPACT: Medium
READ TIME: 2 mins
Quality Level Mean [1 – 10]: 8
1. “To identify specific sources of confounding in retrospective observational research studies – and ultimately to support clinician decision making – Jiaming Zeng, a PhD candidate in Management Science and Engineering, teamed up with Shachter, professor Susan Athey of Stanford Graduate School of Business, and Stanford Medical School professor Daniel Rubin and clinical associate professor Michael Gensheimer.”
2. “Zeng and her collaborators used relatively simple machine learning methods to identify clinically meaningful sources of treatment selection bias in the unstructured text portion of patients’ electronic medical records.”
3. “For example, a 2015 retrospective study of treatments for prostate cancer suggested that surgery was better than radiation for patients’ overall survival, but a subsequent RCT showed that survival was actually the same for patients treated with either radiation or surgery.”
4. “Zeng extracted biomedical terms from the prostate and NSC lung cancer patients’ unstructured clinical notes and then used a simple natural language processing technique called “bag of words” to generate a matrix of word frequency counts.”
5. “When Zeng did what was essentially a classic retrospective population-based study of prostate cancer treatments without including her unstructured text, she found that patients treated with radiation or monitoring fared somewhat better than those treated with surgery – a result that differed from the RCT findings.”
Source URL: https://hai.stanford.edu/news/using-clinical-text-combat-selection-bias-medical-research