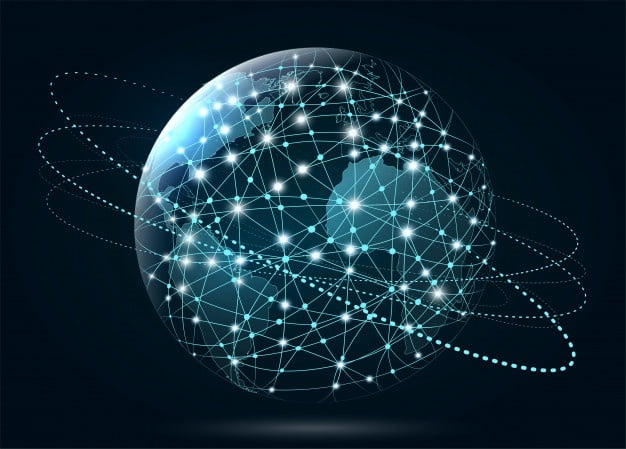
CONTEXT: Whilst this story is not directly related to health data, we think it is worth a read. It feels like we are hearing about new terminology every day, but here’s one that we should be aware of as we move to exploit the benefits of RWD – Data poisoning. Of course people will try to ruin good things, if there is money in it. You can imagine the blackmailing opportunities of holding data to ransom, or it will be poisoned. That the very technology we hope to help us (ML) could be turned to corrupt the data was inevitable, wasn’t it?
IMPACT: High
READ TIME: 3 mins
1. “Data poisoning or model poisoning attacks involve polluting a machine learning model’s training data.”
2. “Other types of attacks can be similarly classified based on their impact:. The difference between an attack that is meant to evade a model’s prediction or classification and a poisoning attack is persistence: with poisoning, the attacker’s goal is to get their inputs to be accepted as training data.”
3. “Data poisoning can be achieved either in a blackbox scenario against classifiers that rely on user feedback to update their learning or in a whitebox scenario where the attacker gains access to the model and its private training data, possibly somewhere in the supply chain if the training data is collected from multiple sources.”
4. “”If your model’s performance after a retraining takes a dramatic hit, whether or not it’s a poisoning attack or just a bad batch of data is probably immaterial and your system can detect that,” Anderson says.”
5. “”A lot of security in AI and machine learning has to do with very basic read/write permissions for data or access to models or systems or servers,” Anderson says.”
Source URL: https://www.csoonline.com/article/3613932/how-data-poisoning-attacks-corrupt-machine-learning-models.html