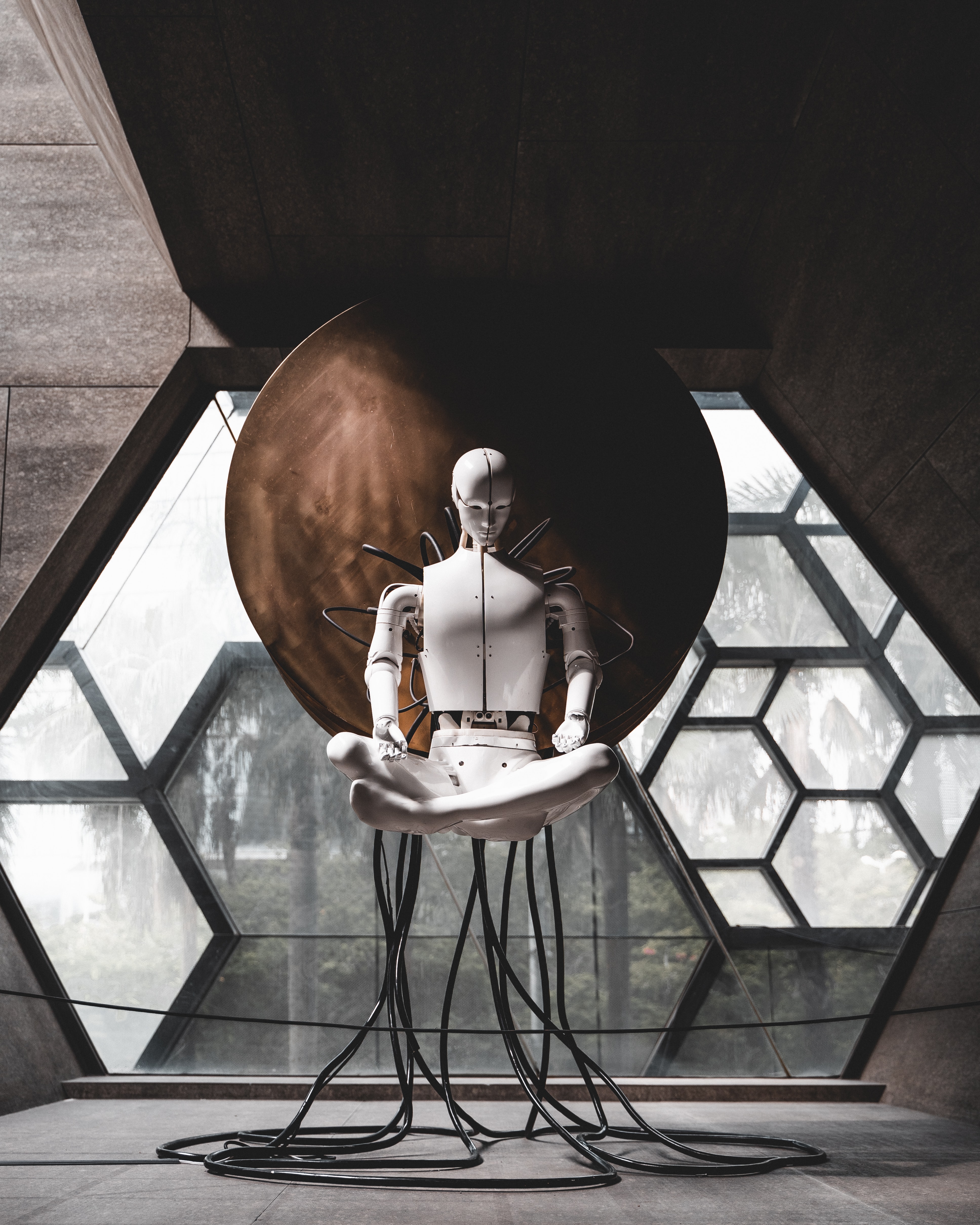
CONTEXT: Peer reviewed article on machine learning (ML) algorithms in an observational schizophrenia study | Dust off your stats textbooks and start googling those ML algorithms to get the most out of this read, but it is well worth the effort | We will be seeing much more of these predictive models utilising ML and across a range of challenging indications like this | “Random Forest Model”…errrr…
IMPACT: High
READ TIME: 10 mins
Quality Level Mean [1 – 10]: 9
1. “In most patients with schizophrenia onset in adolescence, social functioning is severely affected and progressively declines as the number of acute episodes increases.3,4. Many studies have focused on factors associated with social functioning and previously identified potential predictors including sociodemographic factors, illness duration, Positive and Negative Symptom Scale (PANSS) scores, cognition, antipsychotic use and others.”
2. “Of these predictors, patients with a poor functional prognosis were found to be older, have a longer illness duration, have more severe psychiatric symptoms, have worse cognitive functioning, be unemployed, be poorly educated, and be male.5–14 However, the comprehensive effect of these factors on social functioning is still unclear since individual patients with schizophrenia demonstrate great heterogeneity in disease conditions, adverse drug reactions, family economy and other characteristics; and predicting their social functional outcomes using traditional methods is difficult.”
3. “Therefore, based on our observational study on Chinese schizophrenia patients taking atypical antipsychotics (AAPs), we aimed to establish a predictive model of social functional improvement using the baseline and 3-month follow-up information of patients with AAP monopharmacy.”
4. “The PSP score can be divided into 10 levels using every 10 points to represent different degrees of dysfunction, and the minimum detectable change caused by nonrandom variables in continuous measurements of the PSP was 10.7 points.22 In this study, the positive social functional outcome of machine learning was defined as an increase of ≥10 points in the PSP score after treatment.”
5. “The predictive variables were from a series of clinically available information in the SALT-C study, including demographics, psychiatric symptoms (PANSS, the Calgary depression scale for schizophrenia [CDSS], and clinical global impression-schizophrenia [CGI-S]), antipsychotic use, treatment compliance (medication satisfaction questionnaire [MSQ], drug attitude inventory [DAI-10] and subjective well-being under neuroleptics [SWN]), the tolerability of AAPs (Simpson-Angus scale [SAS], Barnes akathisia [BARS], abnormal involuntary movement scale [AIMS], electrocardiograph [ECG] and serum prolactin), comorbidities and concomitant medication use.”